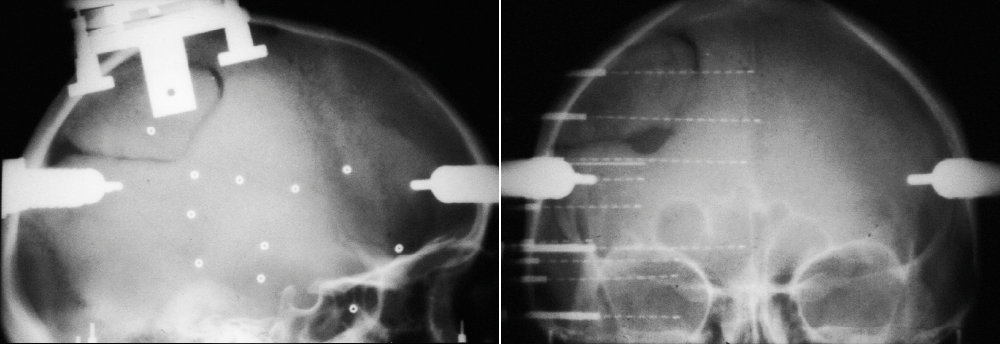
Intracortical electrodes in humans and deep learning for vision
By now we can say that there is a long tradition of comparing the activity in the human brain, especially along the ventral stream, with the activations of artificial neurons in deep convolutional neural networks. It was first attempted around 2015 and since then has been tried using various neuroimaging modalities, deep learning architectures, and comparison metrics (Representational Similarity Analysis leading the way).
However, most of the studies are limited by the neuroimaging method they use: low temporal resolution of fMRI does not allow to measure fine temporal dynamics of human response to visual stimuli, while noise and aggregate nature of EEG and MEG does not let us peek deeper into the cortex and have precise information about the anatomical locations of the signal.
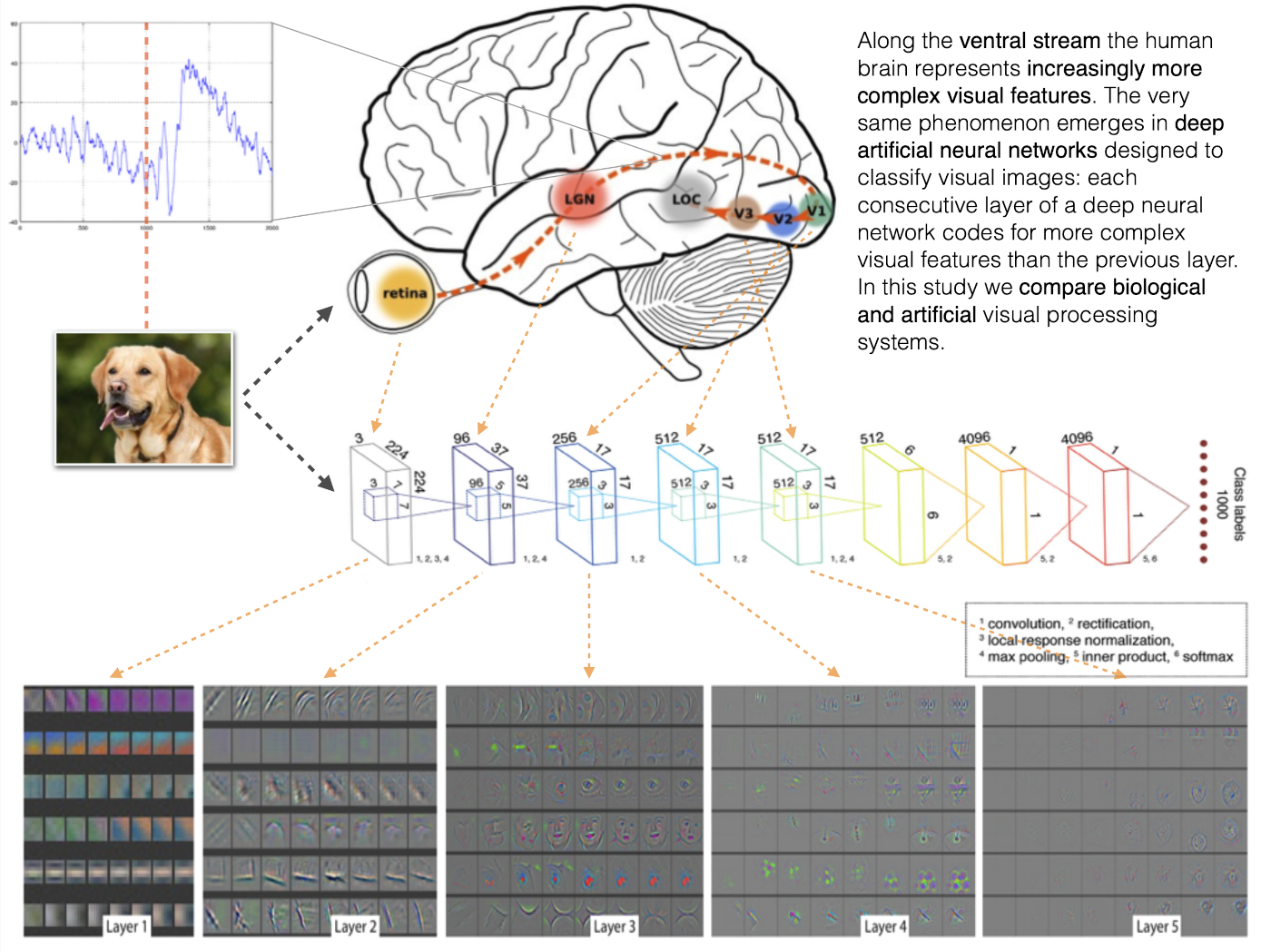
In this project we had access to a unique data collected from a 100 human participants who had electrodes implanted in their cortical and sometimes subcortical regions.
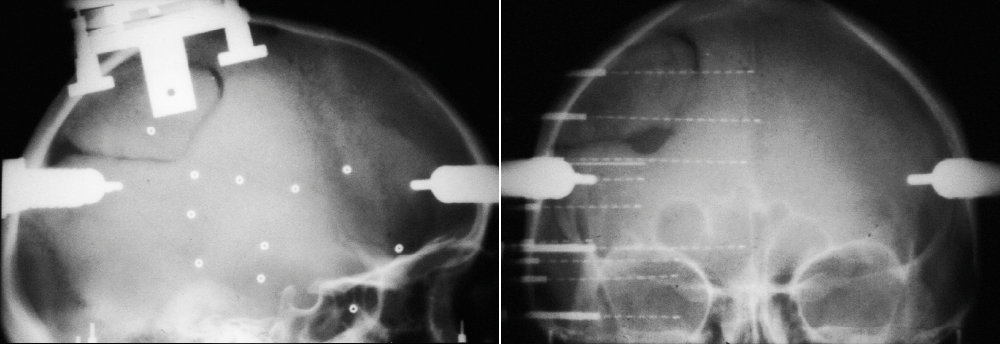
This gave us a unique ability to both know the anatomical location of the recording site, and have access to electrophysiological signal of high temporal resolution. We knew from previous studies that there is a curious alignment in representational similarity between brain areas along the ventral stream and layers of a deep convolutional neural network. Now we wanted to find out where, when, and at which frequencies of neural oscillation this alignment is the strongest.
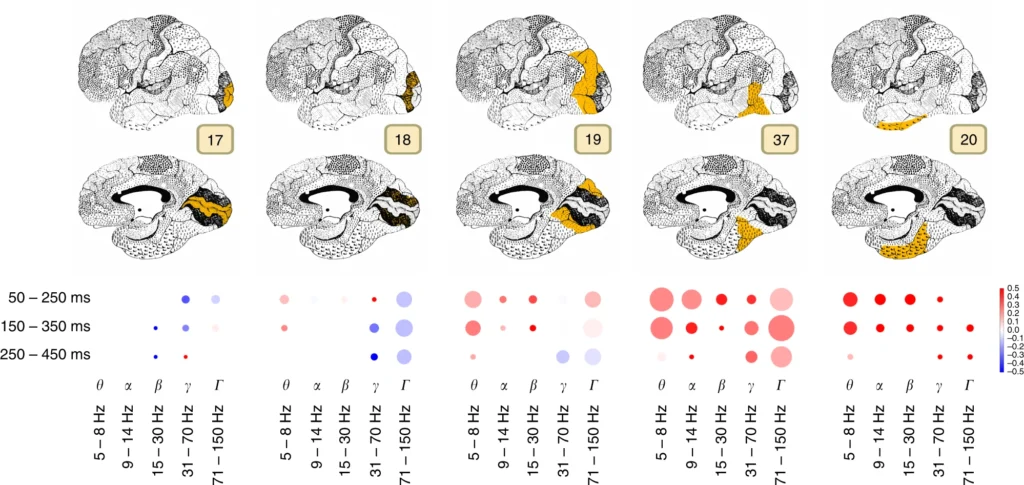
From this work we have obtained an interesting map presented on the image above, summarising when after stimulus onset and at which frequencies the brain signal is correlated with the layers of a deep convolutional neural network and how complex the visual representation that brings this correlation forward is.
References
Kuzovkin, I., Vicente, R., Petton, M. et al. Activations of deep convolutional neural networks are aligned with gamma band activity of human visual cortex. Commun Biol 1, 107 (2018). https://doi.org/10.1038/s42003-018-0110-y
No comments yet.